Bayesian Networks in Forestry: A Comprehensive Overview
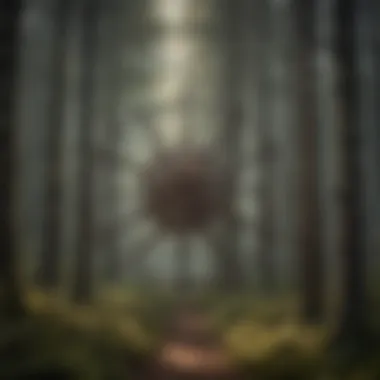
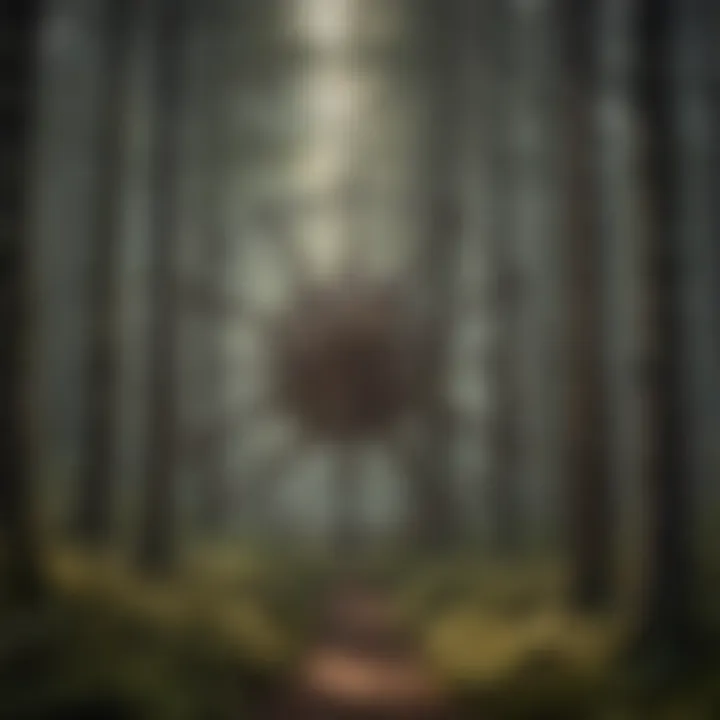
Intro
Bayesian networks are powerful tools in many domains, including forestry. Their probabilistic nature allows for modeling complex ecological systems, with various interrelated factors. This overview will explore how these networks can be applied to enhance forest management practices and woodland stewardship strategies. With a focus on data integration and informed decision-making, we will delve into the fundamental concepts that underpin these methodologies.
Overview of Forestry Practices
Definition of Forestry
Forestry refers to the art and science of managing forests, tree plantations, and related resources. It encompasses a wide range of activities, such as planting, growing, maintaining, and harvesting trees. Additionally, forestry involves managing forest ecosystems to optimize environmental, economic, and social benefits. This discipline is vital for sustaining healthy forests that provide numerous ecosystem services.
Importance of Forestry in Ecosystem Management
Forestry plays several crucial roles in ecosystem management. Healthy forests contribute to biodiversity, carbon storage, and soil protection. They offer habitats for various species and regulate water cycles. Furthermore, forestry practices can help combat climate change by sequestering carbon dioxide. Thus, effective management is necessary to maintain these essential services and promote sustainability within forested regions.
Sustainable Forest Management
Principles of Sustainable Practices
Sustainable forest management (SFM) aims to balance ecological, economic, and social goals. It emphasizes the need to preserve forest ecosystems while allowing for resource use. Key principles include:
- Long-term viability: Ensuring that forest management decisions do not compromise future generations.
- Community involvement: Engaging local communities to harness traditional knowledge and promote shared responsibility.
- Ecosystem health: Prioritizing biodiversity and maintaining healthy habitats.
Techniques in Sustainable Management
To implement SFM effectively, various techniques can be employed. These include:
- Selective logging: Reduces impact on ecosystems by allowing some trees to remain for regeneration.
- Reforestation: Involves planting new trees to restore deforested areas.
- Forest certifications: Programs such as the Forest Stewardship Council (FSC) encourage responsible forest management practices.
Woodland Stewardship Strategies
Importance of Woodland Stewardship
Woodland stewardship is crucial for maintaining healthy ecosystems and ensuring the sustainability of forest resources. By implementing effective stewardship strategies, landowners can significantly contribute to environmental protection and community well-being. This approach fosters a sense of connection between the land and its stewards.
Best Practices for Landowners
Landowners can adopt several best practices to enhance woodland stewardship:
- Conduct regular assessments: Evaluate forest health and biodiversity to identify areas needing attention.
- Collaborate with local organizations: Partner with forestry experts and community groups for knowledge sharing.
- Implement conservation practices: Protect water resources, manage wildlife habitat, and control invasive species.
By integrating these principles and practices, landowners can play an active role in sustainable forest management while also benefiting from the resources their woodlands offer.
Effective woodland stewardship leads to healthier ecosystems and more resilient forest landscapes.
Prelims to Bayesian Networks
Bayesian networks represent a compelling approach to modeling uncertainty in various fields, including forestry. Their capacity to integrate diverse data sources and represent complex relationships is particularly useful for forestry professionals and environmental researchers. Understanding how to apply Bayesian networks can enhance decision-making processes, inform risk assessments, and ultimately support sustainable forestry practices.
Definition and Purpose
A Bayesian network is a graphical model that depicts a set of variables and their conditional dependencies via a directed acyclic graph. The nodes in the graph represent variables, while the edges signify the probabilistic dependencies between them. The primary purpose of Bayesian networks is to model uncertainty, allowing researchers to reason about uncertain information using known probabilities.
The integration of Bayesian networks into forestry management provides several benefits:
- Risk Assessment: They enable practitioners to evaluate and manage risks associated with forest ecosystems.
- Data Fusion: The model allows for the incorporation of multiple types of data, enriching the analysis.
- Improved Predictions: Decision-making can be enhanced through probabilistic predictions based on existing knowledge.
By constructing and analyzing these networks, users can gain insights into system dynamics that are often obscured in traditional analytical methods.
Historical Context
The development of Bayesian networks stems from concepts in statistics and probability theory. Their roots can be traced back to the work of Thomas Bayes in the 18th century, who introduced the idea of updating beliefs in light of new evidence. However, the practical application of these concepts in graphical models emerged later.
In the 1980s, the integration of graphical models with Bayesian inference was formalized, which allowed researchers to visualize and reason about complex, uncertain systems. The decade saw significant advancements in computational algorithms, making it feasible to apply Bayesian methods to a variety of real-world problems.
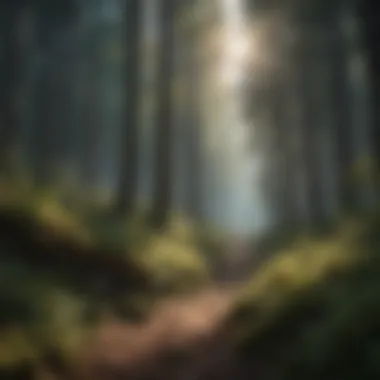
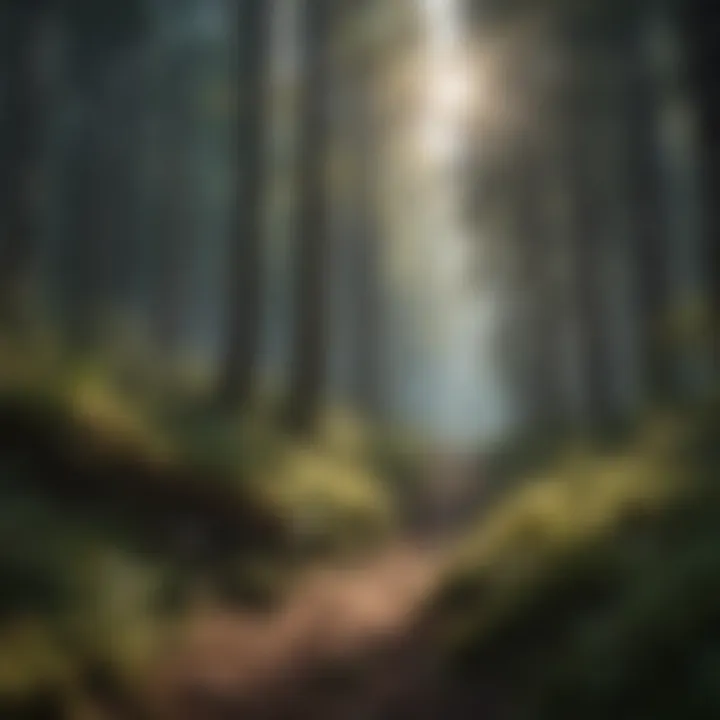
Since their inception, Bayesian networks have been applied across diverse domains, including artificial intelligence, bioinformatics, and economics. In forestry, the adoption has been slower but steadily growing as the advantages of this approach become apparent.
By understanding the historical evolution of Bayesian networks, one can appreciate their potential for addressing contemporary challenges in sustainable forestry management. This background sets the stage for exploring the complex, dynamic interactions that define forest ecosystems.
Fundamental Components of Bayesian Networks
Bayesian networks are built on foundational elements that determine their functionality and application. Understanding these fundamental components is crucial for anyone looking to implement these models in forestry. The key aspects include nodes and variables, edges and conditional dependencies, and probabilistic graphs. Each of these components plays a distinct role in how Bayesian networks operate and contribute to effective decision-making and analysis in forestry management.
Nodes and Variables
In the realm of Bayesian networks, nodes represent random variables. These variables may correlate with various aspects of forestry, such as tree growth rates, species distributions, or environmental factors. Each node can take on a range of values, which represent different states or conditions.
The nature of these variables can be discrete or continuous. Discrete variables might represent categorical data, like the presence or absence of a specific species, while continuous variables can indicate measurements, such as rainfall amounts. Understanding the behavior of these variables is essential because they influence the network’s overall predictions and inferences.
Edges and Conditional Dependencies
Edges in a Bayesian network illustrate the relationships or dependencies between nodes. A directed edge from one node to another implies that the first variable influences the second. This captures the essence of probabilistic reasoning, where the state of one variable can affect the state of another.
Conditional dependence is a key concept here. It provides insight into how knowledge of one node (for instance, soil composition) can affect beliefs about another node (like tree health). Understanding these conditional dependencies allows forestry professionals to ascertain which factors are critical in predicting outcomes and can help guide interventions effectively.
Probabilistic Graphs
At its core, a Bayesian network is a type of probabilistic graph. This means it defines how probabilities are structured among the set of variables represented. Each node is associated with a conditional probability distribution (CPD) that quantifies the effect of its parent nodes. These distributions can be derived from data collected in the field or defined through expert opinion.
The beauty of probabilistic graphs lies in their ability to leverage uncertainty. Given the complex nature of ecological systems, where many variables interact in unpredictable ways, Bayesian networks enable practitioners to incorporate uncertainty directly into their models. This aspect is especially valuable in forestry, where decision-making often occurs under conditions of incomplete or imperfect knowledge.
Understanding the fundamental components of Bayesian networks is pivotal to their effective application in forestry, allowing for sophisticated modeling of ecological interactions and facilitating informed decision-making.
Constructing Bayesian Networks
Constructing Bayesian networks is an essential step in harnessing the power of these probabilistic graphical models for ecological applications, especially in forestry. This process lays the foundation for effective decision-making based on probabilistic reasoning. The construction involves several key components: data collection, model development, and validation. Each of these steps plays a crucial role in ensuring that the resulting network accurately represents the relationships and uncertainties inherent in forestry data.
Data Collection Strategies
Data collection is the first step in building a Bayesian network. Proper data is critical as it feeds into the model, shaping its structure and its ability to make predictions. In forestry, various sources of data can be utilized, including:
- Field Surveys: Direct observations of forest conditions help in collecting reliable information regarding species distribution, health indicators, and environmental factors.
- Remote Sensing: Technologies like satellite imagery can provide large-scale, real-time data about forest cover and land use changes.
- Historical Data: Existing databases can help understand trends and long-term changes in ecosystems, contributing to a better predictive model.
Choosing the right data collection methods depends on the specific objectives of the study and the available resources. It is essential to ensure that the collected data is both relevant and consistent, as inaccuracies can lead to flawed models.
Model Development Process
Once the data is collected, the next step is developing the Bayesian network model. This is where the relationships between variables are quantified using conditional probabilities. Key considerations during this phase include:
- Defining the Structure: The model must accurately represent the dependencies among different variables. This often involves consultation with forestry experts to ensure ecological realism.
- Specifying Conditional Probabilities: Data collected from various sources needs to be translated into probabilistic terms. This is usually done through statistical methods, expert judgment, or a combination of both.
- Software Tools: Several software options such as Netica or Hugin can facilitate the model-building process. The chosen tool should support the intended complexity of the model and allow for adjustments as new data becomes available.
This step requires collaboration among various stakeholders including ecologists, data scientists, and decision-makers to ensure that the model is robust and practical for real-world applications in forestry.
Validation Techniques
Validation is a critical step in the construction of Bayesian networks. This process ensures that the model's predictions are reliable and applicable in decision-making contexts. Some effective validation techniques include:
- Cross-Validation: This method involves dividing the data into subsets. The model is trained on some of these subsets while being tested on others, allowing for assessment of its predictive capability.
- Sensitivity Analysis: This examines how changes in input variables affect the output predictions. It helps in understanding which variables have the most influence and ensures that the model behaves as expected.
- Comparison with Existing Models: Aligning the new model against established models helps in evaluating its accuracy and relevance. Any discrepancies can prompt further refinements in the structure or probabilities.
Effective validation leads to greater confidence in the model's capacity to inform forestry practices. It also helps communicate the model's reliability to stakeholders who may rely on its outputs for decision-making.
By carefully navigating the stages of data collection, model development, and validation, forestry professionals can construct Bayesian networks that significantly enhance sustainable forest management.
Applications of Bayesian Networks in Forestry
Bayesian networks serve as a powerful tool in forestry management. Their ability to encapsulate complex relationships among various ecological variables makes them indispensable for multiple applications. By utilizing these networks, forestry professionals can enhance their understanding of environmental systems, ultimately leading to more informed decision-making. This section will explore key applications, focusing mainly on risk assessment and management, species population modeling, and ecosystem health monitoring.
Risk Assessment and Management
Effective risk management is crucial in forestry to mitigate threats like fire, pests, and disease. Bayesian networks facilitate this by quantifying risks based on available data. They allow experts to model the probability of adverse events under certain conditions, giving a clearer picture of potential outcomes.
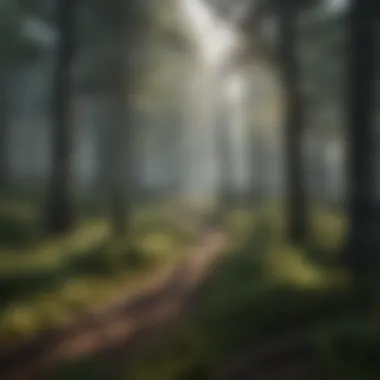
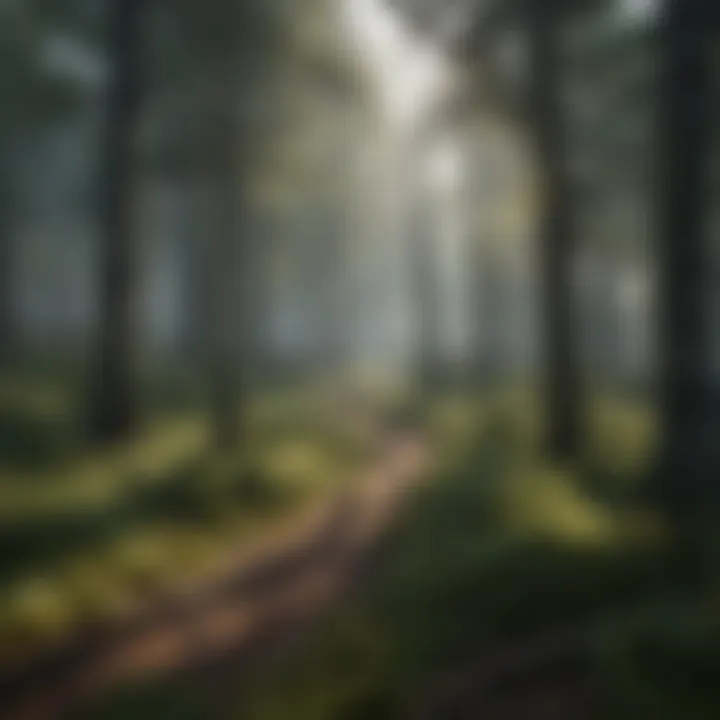
For instance, when assessing the risk of forest fires, these networks can account for various factors such as temperature, humidity, and vegetation types. The interaction between these variables is represented graphically, making it easier to identify critical risk areas. By simulating different scenarios, forestry managers can develop strategies tailored to reduce risks and allocate resources more efficiently.
Species Population Modeling
Understanding species populations is essential for conservation efforts in forestry. Bayesian networks can represent the complexities of population dynamics, incorporating factors like habitat conditions, predation, and human impact.
For example, in studying the population of a specific bird species within a forest, the network can include variables such as food availability, climate variations, and nesting sites. With these factors incorporated, decision-makers can assess how changes in one element impact the overall population. This predictive capability supports conservation programs by targeting specific interventions that can stabilize or increase vulnerable populations.
Ecosystem Health Monitoring
Monitoring ecosystem health is paramount for ensuring sustainable forestry practices. Bayesian networks provide a framework for evaluating multiple indicators of ecosystem vitality, such as soil quality, tree growth rates, and biodiversity metrics.
Utilizing a Bayesian approach allows for the integration of various data sources, including satellite images and on-ground surveys, into a cohesive model. For example, a forest's health can be monitored by analyzing links between tree health and surrounding conditions, such as moisture levels and nutrient availability. This approach aids in identifying trends and potential threats, enabling proactive measures to foster ecosystem resilience.
In this dynamic environmental landscape, incorporating Bayesian networks into forestry practices not only augments understanding but also aids in developing strategic initiatives that align with conservation goals.
Case Studies of Bayesian Networks in Forestry
Case studies provide practical insights into theoretical concepts and demonstrate the real-world application of Bayesian networks in forestry. They enable researchers and practitioners to see evidence of this methodology effectively managing various forestry challenges. Through well-documented examples, stakeholders can understand the specific behaviors and outcomes associated with Bayesian networks, highlighting their utility in forest management and environmental conservation efforts.
Significant benefits of case studies include showcasing the practical application of complex concepts. They also help validate the effectiveness and reliability of Bayesian methods in ecological systems. It fosters further exploration in the intersection of data science and forestry, illuminating potential areas for innovation and application. Through case studies, forestry professionals can gather insights on how to implement these models within their specific contexts, ultimately contributing to better decision-making processes.
Forest Fire Risk Modeling
Forest fire risk modeling using Bayesian networks is a critical application aimed at assessing the probability and impact of wildfires. These models integrate data from various sources, such as weather patterns, vegetation types, and historical fire events, to predict areas at higher risk of ignition and spread. By representing these complex relationships probabilistically, agencies can prioritize resource allocation and implement preventive measures more effectively.
For instance, consider a case where a woodland area is analyzed for fire risk. By employing Bayesian networks, foresters can incorporate real-time weather data and other dynamic factors. The outcomes not only indicate risk zones but enable policymakers to simulate different scenarios. As a result, the model can help in formulating strategies for evacuation plans or controlled burns based on the forecasted conditions.
Moreover, using Bayesian networks allows for continuous updates and learning as new data becomes available. This adaptive capacity enhances the accuracy of predictions and fosters proactive rather than reactive responses to fire threats.
"Bayesian network models provide a structured framework for integrating and analyzing various sources of information relevant to forest fire management."
Biodiversity Conservation Efforts
Bayesian networks play a vital role in biodiversity conservation efforts within forestry management. They facilitate the integration of ecological data to assess species populations and their interactions with environmental variables. By modeling these relationships, practitioners can identify critical habitats, evaluate the risk of extinction, and develop informed conservation strategies.
For example, a case study focusing on a specific threatened species may utilize data on habitat conditions, climate variables, and human activities. The Bayesian network can illustrate how changes in one variable may impact the population dynamics of the species. This insight is crucial for setting conservation priorities and informing resource allocation for habitat restoration.
Additionally, these models can help in evaluating the effectiveness of different management interventions over time. By simulating various conservation strategies, stakeholders can derive insights into the most effective approaches for maintaining biodiversity.
In summary, the application of Bayesian networks in biodiversity conservation emphasizes their capacity to synthesize complex ecological data into actionable insights, thus enhancing conservation initiatives in forestry.
Data Integration and Bayesian Networks
In modern forestry management, the integration of diverse data sources plays a critical role in leveraging Bayesian networks. This section emphasizes the significance of combining multiple streams of data and the methodologies for managing uncertainty, impacting decision-making and ecological analysis. A coherent integration strategy is essential for effective modeling, enabling forestry professionals to paint a complete picture of ecosystems, risks, and management strategies.
Combining Multiple Data Sources
Combining data from various sources can provide a richer, more nuanced view of forestry systems. This process often involves integrating ecological data, remote sensing information, climate datasets, and socio-economic factors. Each type of data source brings unique insights, helping to inform Bayesian models.
Some key benefits of this integration include:
- Holistic Perspective: By pulling from multiple data pools, models can better reflect complex interdependencies within forest ecosystems.
- Enhanced Predictive Power: More data leads to improved accuracy in predictions, aiding in risk assessment and management.
- Localized Insights: Each data source can capture different environmental nuances, making it easier to tailor strategies for specific regions or species.
Challenges arise when dealing with varying data formats, quality, and spatial resolutions. To effectively combine these sources, practitioners often utilize data transformation techniques and standardization methods.
"The credibility of Bayesian networks hinges significantly on the data quality and diversity being utilized."
Thus, careful consideration of the source and nature of data is imperative. Best practices involve developing a protocol to evaluate data reliability and relevance before integrating it into the network.
Handling Uncertainty in Data
Uncertainty is an inherent aspect of ecological data. Variability in measurements, gaps in data collection, and prediction errors can lead to significant challenges when modeling forest dynamics with Bayesian networks. However, Bayesian methodologies are particularly adept at handling uncertainty by using probabilistic frameworks, which allows for a more rigorous analysis of potential outcomes.
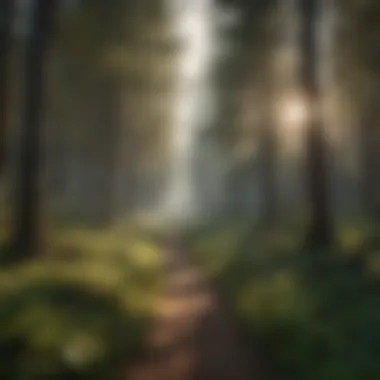
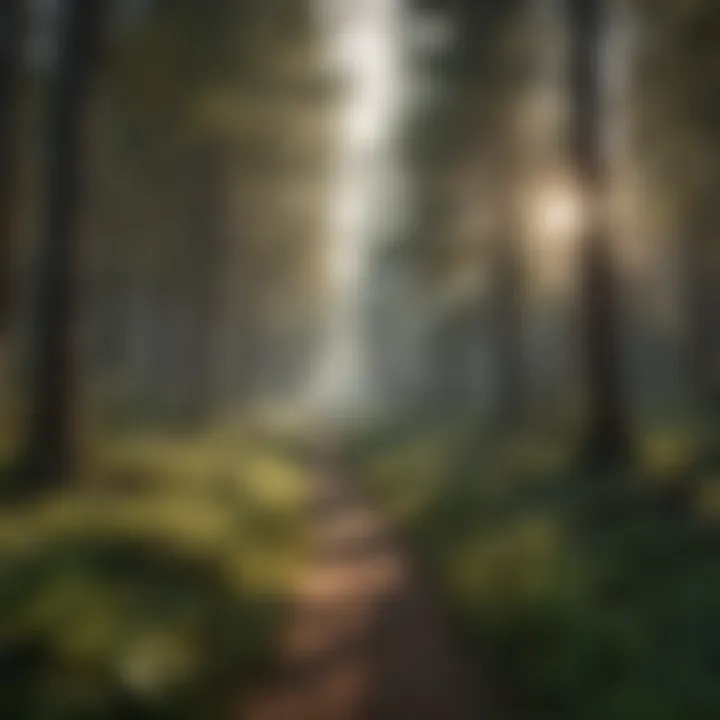
Handling uncertainty involves several strategies, including:
- Probabilistic Modeling: This approach allows practitioners to encapsulate uncertainty directly in the model parameters, adjusting the precision as more data becomes available.
- Sensitivity Analysis: Analyzing how sensitive the model outcomes are to changes in input data can reveal potential weaknesses and guide data collection efforts.
- Incorporating Expert Knowledge: When empirical data is lacking, input from subject matter experts can help inform model structures and assumptions, providing a qualitative layer of understanding.
By actively engaging with uncertainty rather than avoiding it, forestry professionals can enhance model robustness. In turn, this leads to more informed forest management strategies, contributing to sustainable practices and long-term ecological health.
Future Directions and Innovations
The exploration of future directions and innovations in Bayesian networks within forestry is crucial. As our understanding of ecological systems deepens, the need for refined modeling approaches increases. The integration of Bayesian networks with emerging technologies presents opportunities for enhanced decision-making and sustainability efforts in forestry management. This section will discuss significant advancements particularly related to computational techniques and the integration with machine learning.
Advancements in Computational Techniques
Computational techniques play a vital role in the development and application of Bayesian networks. As data grows in volume and complexity within the forestry sector, the sophistication of computational models becomes essential. Recent advancements in computational power allow researchers to analyze larger datasets effectively.
Key advancements include:
- Parallel Processing: This technique enables simultaneous computations, thereby reducing the time required for model training and evaluation.
- Approximation Algorithms: These algorithms, such as Markov Chain Monte Carlo (MCMC), facilitate inference in complex models.
- Cloud Computing: Utilizing cloud-based platforms offers scalable solutions for data storage and processing, accommodating extensive ecological datasets.
The implementation of these advancements not only improves efficiency but also enhances the accuracy of predictions made by Bayesian networks. As a result, forestry managers can make well-informed decisions quickly, considering diverse factors that affect forest health and management strategies.
Integration with Machine Learning
The synergy between Bayesian networks and machine learning is one of the most promising future directions. Machine learning techniques can augment the functionality of Bayesian networks by providing better mechanisms for learning from data and making predictions.
Some important aspects of integration include:
- Enhanced Predictive Models: Machine learning algorithms can analyze vast amounts of historical data and identify patterns, which can then inform the Bayesian models.
- Dynamic Updating: Integrating machine learning allows Bayesian networks to adaptively update their parameters as new data becomes available, enhancing their relevance and accuracy.
- Automated Feature Selection: Machine learning techniques can assist in identifying the most influential variables affecting ecosystems, streamlining the model-building process in Bayesian networks.
The collaboration between these two fields opens new pathways for ecological assessments and sustainable forestry practices. Forest managers equipped with these advanced tools can predict outcomes related to species population dynamics or assess the risk of forest fires with greater precision.
This integration is not merely a theoretical exploration; it is actively shaping the methodologies of contemporary ecological research and practical forestry management.
Challenges in Implementing Bayesian Networks
The implementation of Bayesian networks in forestry presents several challenges that need careful consideration. Understanding these challenges is crucial for forestry professionals and researchers aiming to utilize this powerful tool effectively. It not only indicates the limitations of the application but also underscores the necessary strategic approaches to mitigate these issues. Through this exploration, we can better appreciate how to maximize the potential benefits of Bayesian networks while addressing inherent difficulties.
Data Limitations
Data limitations significantly impact the performance and reliability of Bayesian networks. Accurate data is crucial for constructing effective models. However, several factors can limit data availability.
- Insufficient Quality: Often, the data collected in forestry might lack precision. This can stem from various sources such as faulty measurement tools or human errors during data collection.
- Incompleted Data: Historical records may be incomplete or not well-maintained. Missing information can lead to biased analyses.
- Temporal and Spatial Variability: Forest environments are complex and ever-changing. Data collected might not represent current conditions accurately due to factors like seasonal changes or disturbances.
These limitations can lead to flawed models, resulting in poor forecasting and inadequate decision-making. Therefore, developing strategies to enhance data collection and quality is imperative.
Interpretability of Models
Interpretability refers to the clarity with which results from Bayesian network models can be understood. This is especially important in forestry, where decisions based on model predictions can have significant ecological and economic impacts. However, interpretability can be a major hurdle for practitioners.
- Complex Structures: Bayesian networks may involve numerous variables interacting in complex ways. This complexity can make it challenging for decision-makers to draw meaningful insights from the models.
- Layman Understanding: Many forestry professionals might lack advanced statistical and modeling knowledge. Thus, presenting model outcomes in a way that is readily comprehensible to non-experts is essential.
- Communication of Uncertainty: Bayesian methods are grounded in probability, which inherently includes uncertainty. Effectively communicating this uncertainty and its implications on decision-making requires skillful presentation.
Addressing these challenges is paramount. Innovations such as improving visualization tools and providing tailored training can enhance the interpretability of models. This ensures that end-users can apply findings confidently and effectively in managing forestry resources.
Effective interpretation empowers forest managers to use Bayesian networks more beneficially, leading towards sustainable practices and informed decisions.
Closure and Summary
In this part of the article, it is essential to emphasize the significance of Bayesian networks in the field of forestry. These networks provide a novel approach to understanding complex ecological relationships and decision-making processes. They allow practitioners to make informed choices based on probabilistic reasoning, which is crucial for effective forest management. The case studies presented earlier highlight how these models can lead to better outcomes in species management and ecosystem health monitoring.
Bayesian networks also facilitate the integration of diverse data sources. This ensures that decision-makers have access to comprehensive information, reflecting the complexity of environmental systems. The interplay between uncertainty and data can be streamlined using Bayesian methodology, presenting a clearer picture for practitioners.
The synthesis of this information underscores the relevance of Bayesian networks in promoting sustainable practices in forestry. As the industry faces various challenges, such as climate change and habitat degradation, these networks could lead to more resilient and informed forest management strategies.
Key Takeaways
- Bayesian networks enhance the ability to model and analyze ecological systems.
- They provide insights that improve decision-making in forestry management.
- Successful case studies exemplify the practical benefits of these networks in addressing ecological challenges.
Recommendations for Practitioners
- Embrace data integration: Utilize multiple data sources to build more robust models. This provides a more comprehensive understanding of the ecological landscape.
- Invest in training: It is crucial for professionals in forestry to understand how to develop and implement Bayesian networks effectively. Continued education is key.
- Adopt probabilistic thinking: Encourage a shift from deterministic methods to probabilistic approaches when assessing risks and outcomes in forest management.
- Collaborate across disciplines: Engage with data scientists and ecologists to explore innovative uses of Bayesian networks. Their insights can enhance model development and applications.
Bayesian networks support strategic planning in forestry, addressing uncertainties while paving the way for sustainable practices. It is a recommended approach for all professionals involved in forest management.